The AI in AEC 2025 conference showcased various technologies, applications, and case studies. Takahiro Morohashi, a researcher at the Kajima Research Institute, introduced an innovative technology that utilizes AI to analyze ambient sound from a job site.
Getting data for situational awareness
AI-powered construction progress monitoring applications require data from the job site to assess the project’s status compared to the schedule. Various methods exist to capture that data, such as IP and mobile cameras, sensors, and manually filled forms.
You can’t easily cover the whole site with stationary cameras. Hence, you need a person or a robot to regularly walk around the site with a 360-degree camera or fly a drone to collect visual information for progress updates. In addition, or as an alternative, you can use sensors and even elevators as data collectors.
Every data capture method has advantages and limitations, but implementing and maintaining them can be expensive on a large construction site.
Ambient sound as a data source
Construction is a noisy business. I live near an extensive greenfield residential development, with one project following another. The thumping sounds of pile drives have become all too familiar, as the ground of the sites is predominantly ancient seabed.
Interior work also generates noise. Most of the work today uses machines that create a particular sound.
Utilizing sound as a data source has certain advantages. Microphones for this purpose are pretty cheap and cover large areas without “seeing” everywhere. Audio data is light compared to video and can be streamed or transmitted quickly to the cloud for AI analysis.
How the model was trained
Morohashi explained how the research team had focused on four sound sources: high-speed cutters, arc welding, power staplers, and construction elevators.
The team trained an AI model using supervised learning by annotating work activities from audio recordings captured in real construction environments. For those interested in the specifics, their deep learning model consists of three layers of Convolutional Neural Networks to analyze sound features (such as spectrograms) and a fully connected layer that classifies the work activity based on those features.
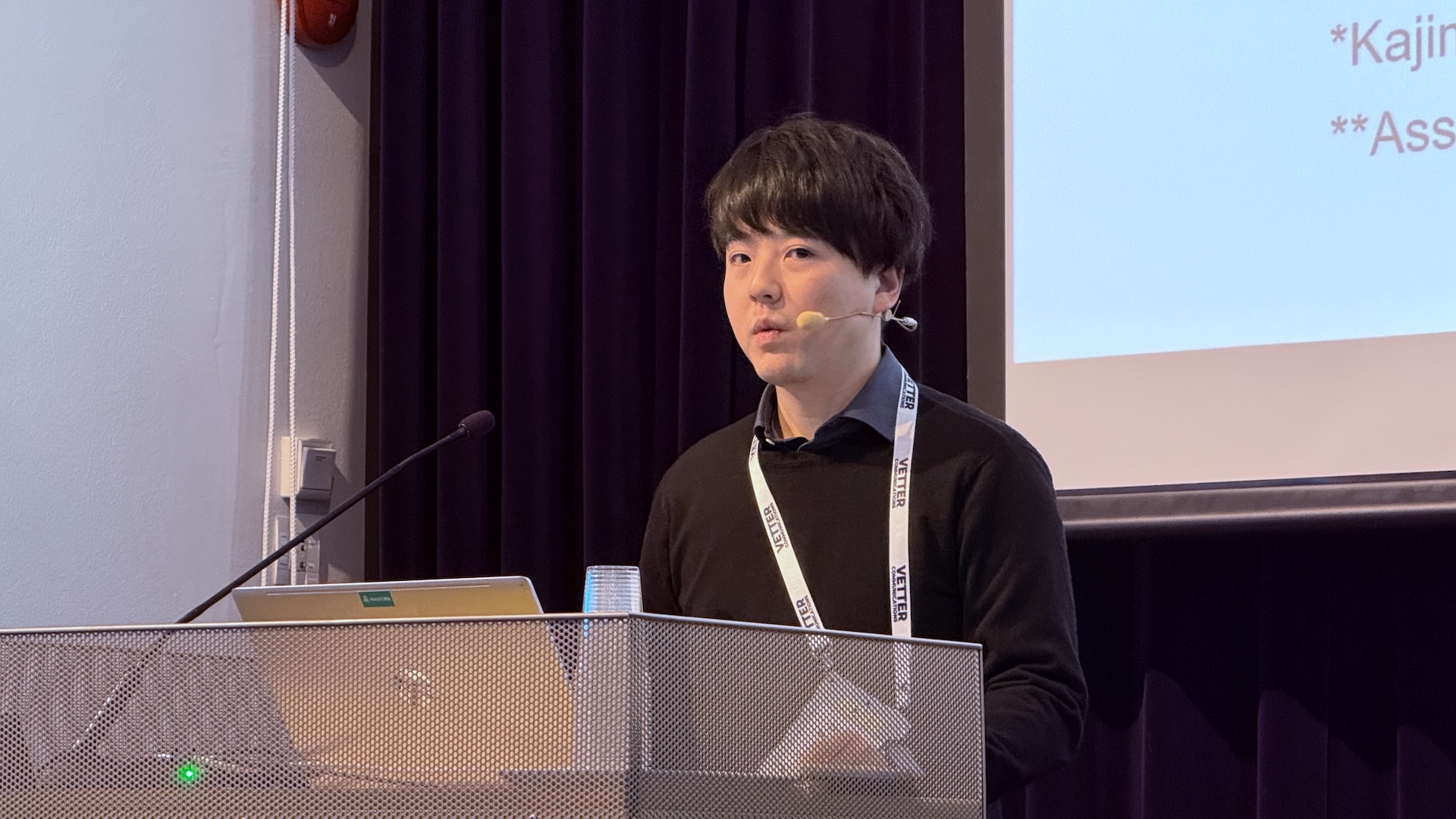
The results
The study included 49,400 sound files, of which 10,100 were labeled sounds.
The AI application performed well. The average F-score (F1-score), which measures a model’s accuracy, was 79%, meaning the AI system could recognize work activities from audio about four out of five times.
Arc welding was the most difficult to detect correctly, possibly because of the audio sampling rate and compression. Its score was just short of 13%.
How does this help in progress monitoring
The identified audio events have a timestamp, which allows them to be automatically placed on a timeline. Because the location of the microphones (e.g., “the third floor”) is known, the activities can be mapped and compared to the work schedule.
This method can transform remote construction progress monitoring as the accuracy of the model improves and more activity types are added.
This was just one example of the numerous exciting AI applications we noted at the AI in AEC conference.
View the original article and our Inspiration here
Leave a Reply