Artificial Intelligence has been in the headlines for the past decade across several disciplines. From building systems to detect breast cancer that outperform expert diagnosticians, to start-ups creating self-driving cars, the AI momentum has built up exponentially. One thing is for sure: AI is here to stay.
Interestingly, even though AI has become a buzzword to market a variety of products and services today, it is often misused. AI is an expansive field of research aiming at creating machines that could simulate human intelligence and behaviour. Contemporary AI is far from achieving this goal.
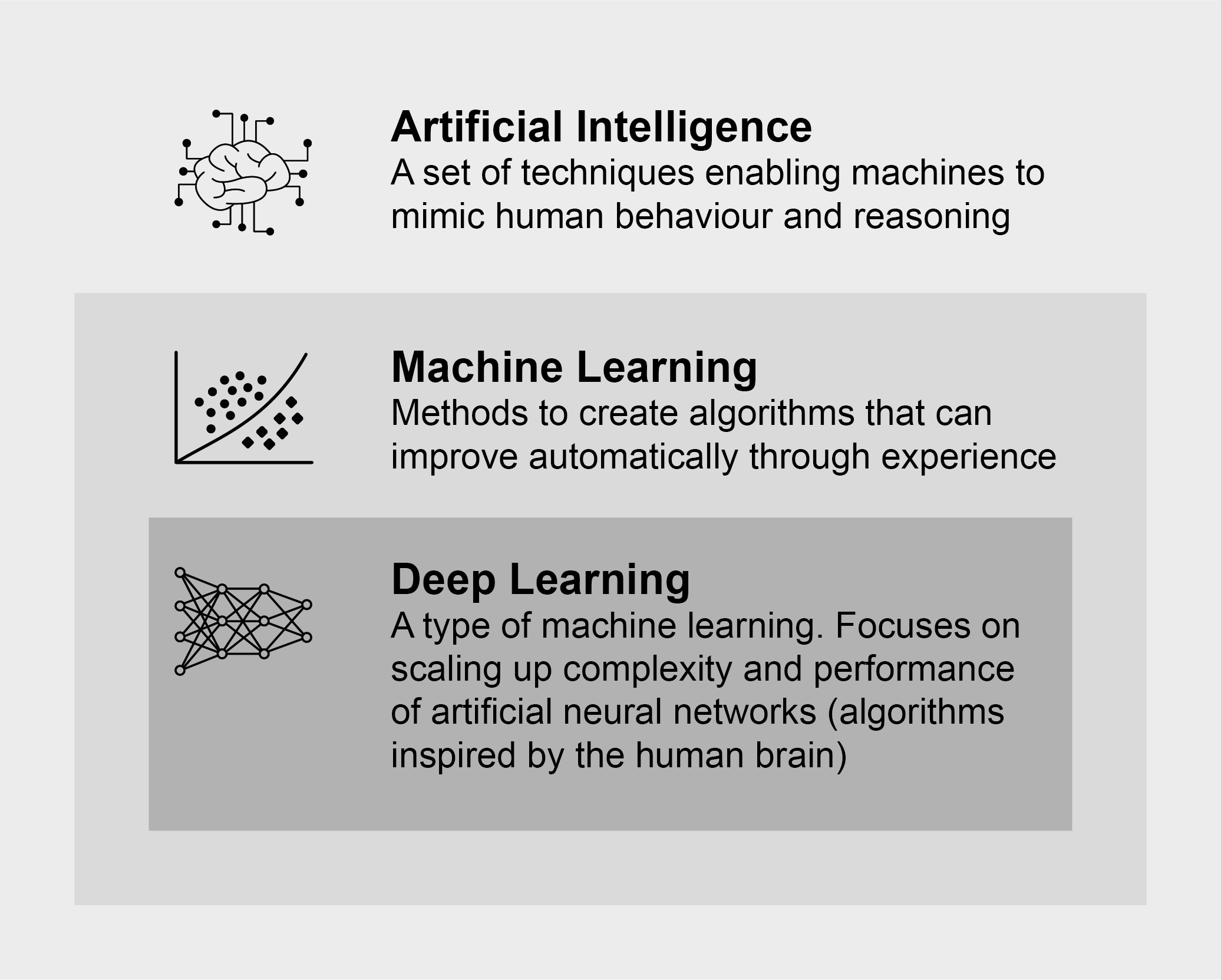
To review more details about the work mentioned in this article and to read an expanded explanation of machine learning, please refer to another article by the authors titled “Artificial Intelligence for the Built Environment” as part of a Springer book Industry 4.0 for the built environment.
Machine learning
The definition of what constitutes intelligence is very elusive and as research progresses, the goalposts are constantly moving. Many of the sub-fields of AI need to be understood better. One of them – which has seen immense progress – is called machine learning (ML). It focuses on building machines that could be trained to improve through experience.
Machine learning attempts to emulate this idea of learning, akin to how humans learn from their external environment; consequently, different algorithms like reinforcement learning, supervised learning, unsupervised learning and intrinsic motivation and exploration attempt to capture and model different nuances of that process.
These algorithms define a “learning process” for a machine, that depends – amongst other things – on the type and amount of data that we have (or not) to train the system with. Data is therefore a common ingredient for all these techniques. In addition, the ability to create copious amounts of data that is relevant to the task at hand or to collate and filter already existing data is one of the most important steps of this process.
Foster + Partners explores ML
The Applied Research + Development group at Foster + Partners has been looking into the potential uses of ML in the AEC industry for some time now. One of the strands we have been exploring is that of surrogate models – also known as approximation models. These ML models can be particularly useful for speeding up some of the slow analytical simulations our designers use.
For example, we trained machine learning models to predict the output of visual graph analysis and connectivity analysis of floor plates. The trained models managed to provide relatively accurate predictions of the results at five hundred times the speed of the respective analytical model, thus redefining the performance-based design process through real-time feedback.
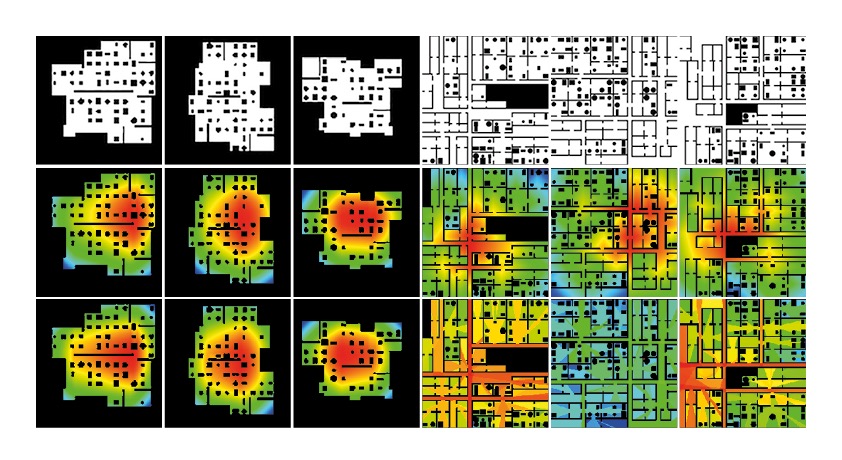
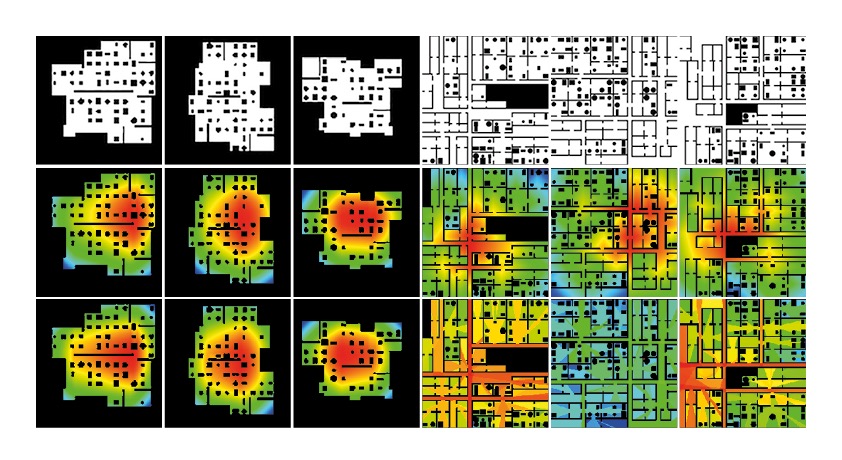
ML models give design suggestions
Another area of interest within the group has been the use of ML design-assist models. These can offer design insights or even suggestions to designers while they are working. One example of such a system was the work we did with Autodesk.
The result of the partnership was a simple application that helps designers predict a layering pattern for thermo-active laminates, which when heated, results in a deformed surface matching their design intent. Not only does this model offer faster results, but it also has the potential of allowing designers to work in a way that was not possible before.
Designing for such a material with complex behaviour could entail hours of manual iterations, attempting to tweak the layering of the laminates to try and get the desired result. By reversing the process, designers can now cut the iteration time drastically and only prototype the samples they are interested in.
ML helps weed through historical design data
In addition to using ML during the design process, we are also investigating augmentation and enrichment pipelines for all types of data that have been collected through the fifty-five years of the practice’s existence.
We use the power of machine learning to help us weed through historical and current data and add a layer of semantics on top, using natural language processing (NLP). Our objective is to make all this data easily accessible for everyone in the office.
We continuously work on identifying interesting design problems and finding the right technology that can facilitate better solutions faster. Being a data-driven organization requires a comprehensive approach, where culture and tools are tailored to deliver high-quality products (designs) at a faster pace than traditional practices.
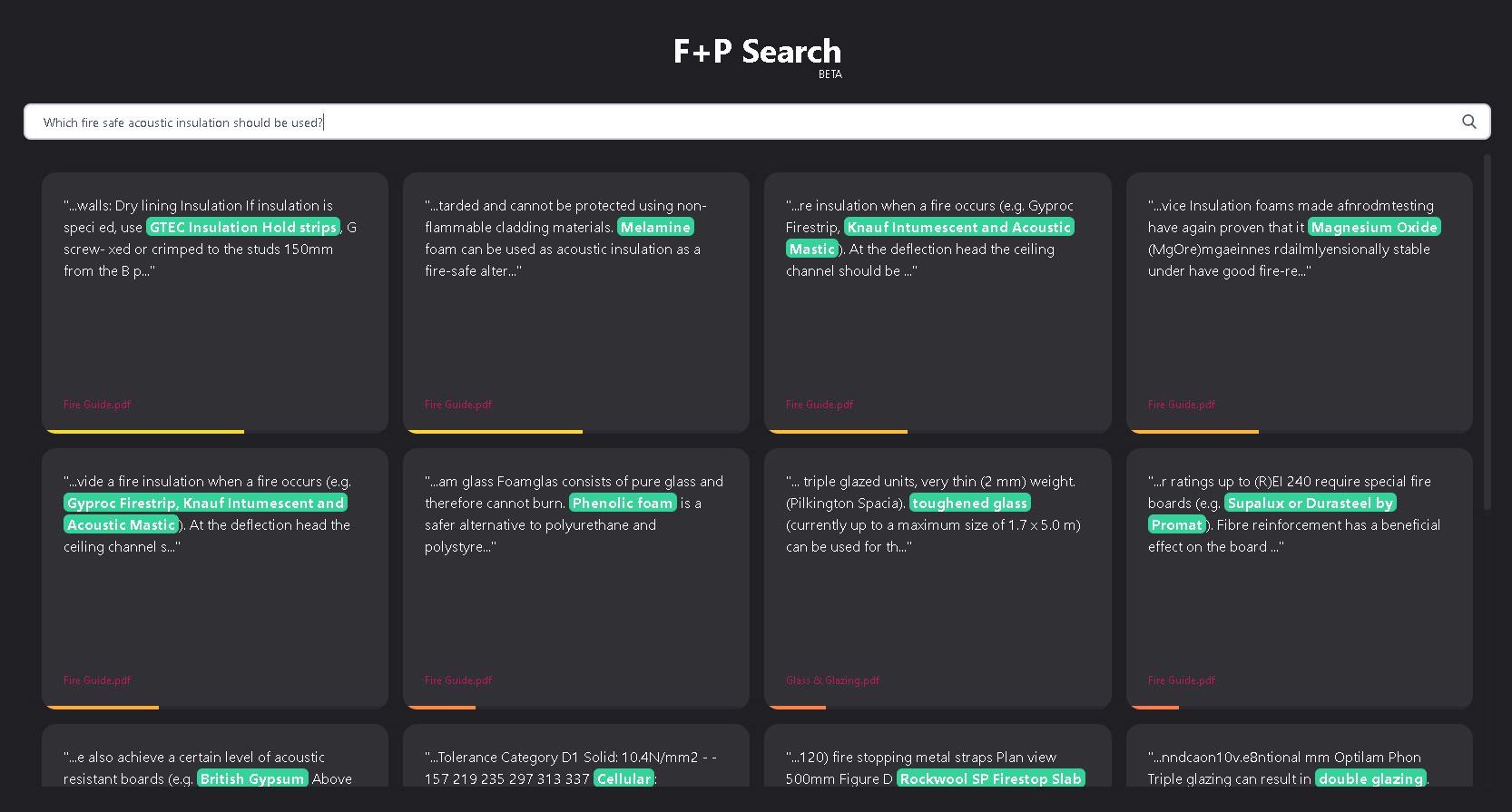
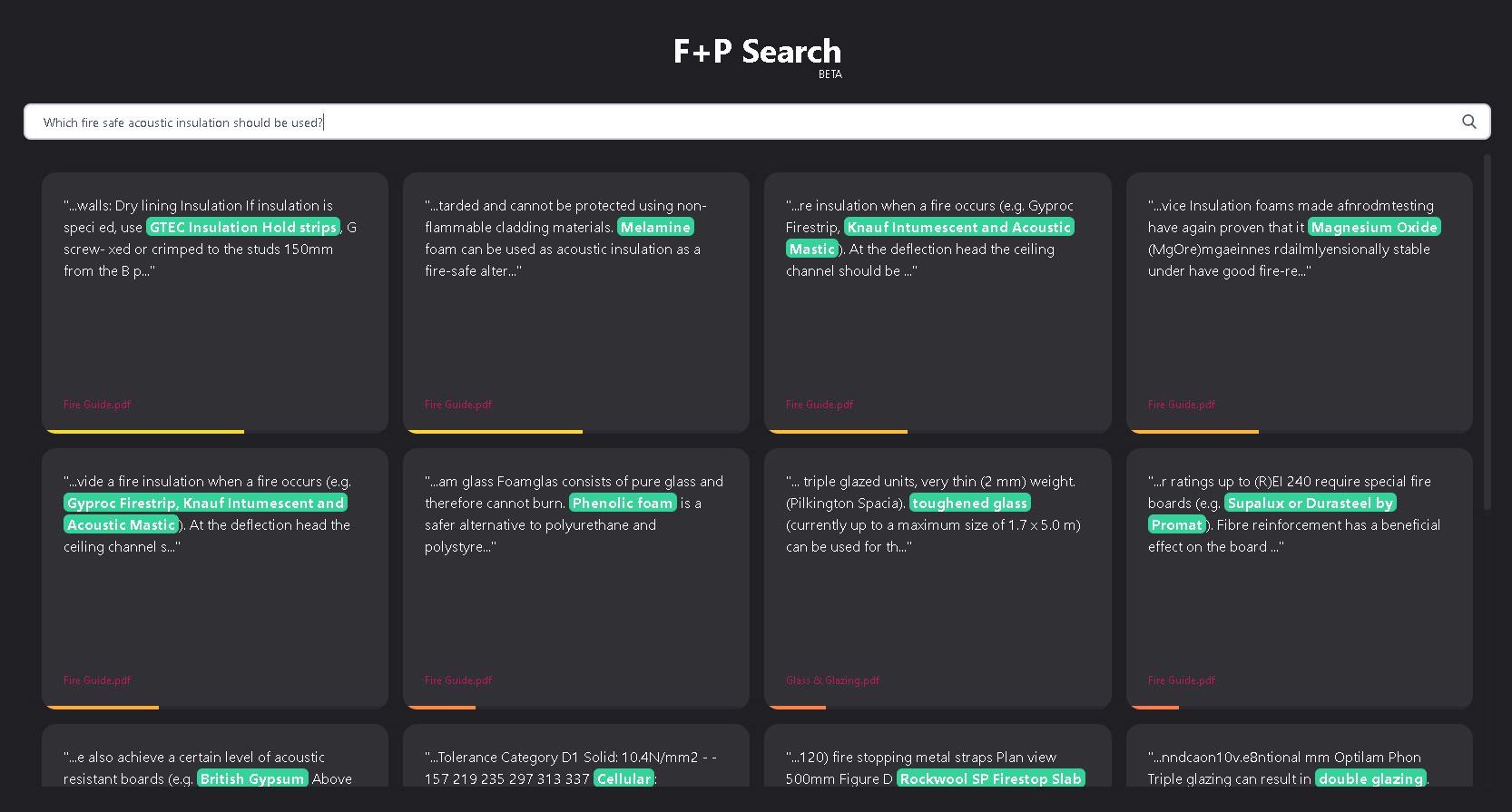
ML techniques integrate within AEC workflows
With the rapid development and rising interest in integrating ML techniques within AEC workflows, we will see the repercussions of this endeavour on performance and efficiency in the coming years. Faster design iterations, integrating heavy analyses in new mediums, speeding up operations that depend on evolutionary optimizations are a few of the areas we see changing and being more accessible to a wider audience.
We are also critically evaluating new job positions that will be in demand in the industry; governance, analysis, and management of data on scales not seen before in our practice; and regulatory and contractual changes in response to new types of data we are handling.
At Foster + Partners, new technologies are perceived not as a threat, but a possibility; to explore new ideas, enhance creativity and constantly optimize and push the boundaries of design. This is the reason AI is a growing part of our research and development output and will continue to be for the near future.
About the authors
Sherif’s position at Foster+Partners’ Applied Research and Development group allows him to work on complex design challenges on a daily basis, utilizing his expertise in geometry optimization, digital fabrication, virtual and augmented reality, and machine learning. He is also an instructor and lecturer at The Bartlett in the MSc. in Architectural Computation and the M.Arch. in Architectural Design courses. For over 12 years, he’s been lecturing, training, and consulting at different universities and firms, discussing and advising on the integration and implementation of different technologies in the design-to-production workflow.
Marcin is currently spearheading the development of Hydra – an in-house cloud platform for computational and performance-driven design. He has also provided expertise in complex geometry, digital fabrication, and machine learning for many high-profile projects all over the world. Marcin graduated both from the Faculty of Architecture of Wroclaw University of Technology, Poland, and The Bartlett School of Architecture, UCL receiving an MSc in Architectural Computation. He is a published author of several research papers as well as a tutor and lecturer at The Bartlett in London.
Martha’s background spans architecture, engineering, and computer science. She has two decades of experience working on projects of all scales and uses. Martha’s work for the Applied Research and Development group incorporates computational design, human-computer interaction, machine learning, and optimization. She has investigated using deep neural networks and genetic algorithms in the design process, aiming to solve problems ranging from passively actuated micromaterials to performance-driven urban layouts.
View the original article and our Inspiration here
Leave a Reply